Pathology, the science of diagnosing disease through the examination of tissues, cells, and bodily fluids, has long been a human-driven domain. Pathologists spend years honing their skills, peering through microscopes to identify cancer cells, infections, or subtle signs of illness in biopsy slides and lab samples. It’s meticulous, time-intensive work—vital to medicine yet often bottlenecked by a shortage of experts and high costs.
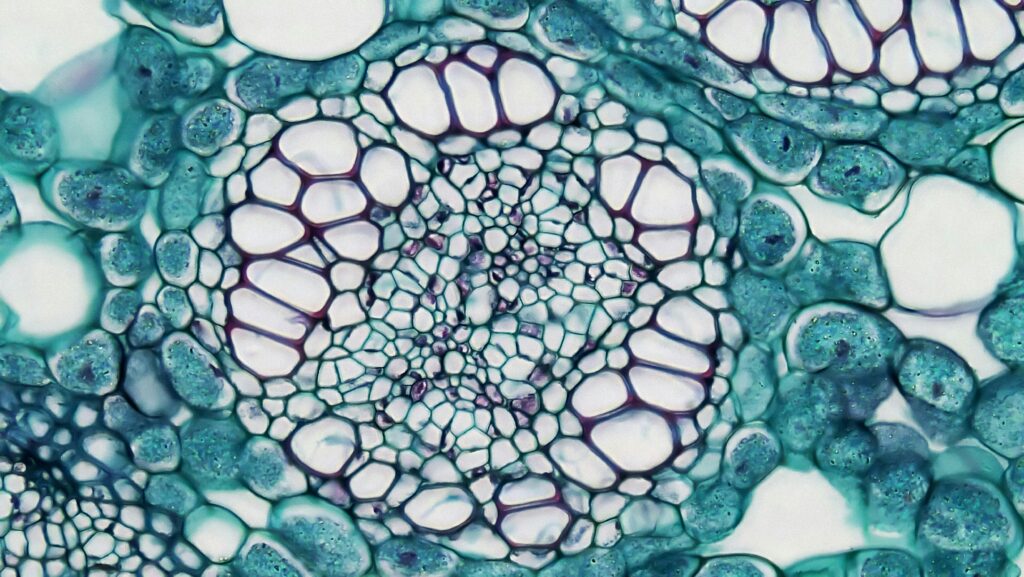
Enter artificial intelligence (AI), which is poised to revolutionize pathology by replacing many traditional human tasks. With its ability to analyze samples at lightning speed and unmatched consistency, AI could not only streamline diagnostics but also bring high-quality pathology services to underserved and economically disadvantaged communities, reshaping healthcare equity in the process.
The Power of Precision and Speed
At its core, pathology relies on pattern recognition—spotting abnormalities in a sea of cells or tissues. AI excels at this. Machine learning algorithms, trained on vast datasets of digitized slides, can identify cancerous cells, inflammatory markers, or infectious agents with a precision that rivals or surpasses human pathologists. For example, a study in Nature Medicine showed an AI system detecting prostate cancer in biopsy samples with 98% sensitivity, outperforming many human experts. Unlike humans, AI doesn’t tire after hours of slide review, nor does it miss subtle clues due to distraction or fatigue.
Speed is another game-changer. A pathologist might take 30 minutes to an hour to thoroughly examine a complex case, such as a breast cancer biopsy, and labs often face backlogs that delay results by days or weeks. AI can process these same slides in minutes—or even seconds—flagging abnormalities for human review or delivering standalone diagnoses. Companies like PathAI and Paige are already deploying such tools, reducing turnaround times dramatically. In a hospital handling hundreds of samples daily, this efficiency could clear backlogs, accelerate treatment, and save lives.
This scalability is key. Where a single pathologist might handle 20-30 cases a day, an AI system could process hundreds, working around the clock. This capacity could transform pathology labs from overburdened hubs into high-throughput diagnostic engines, meeting demand without the need for armies of additional staff.
Democratizing Diagnostics: Pathology for the Underserved
Perhaps the most transformative promise of AI in pathology lies in its potential to bridge the access gap. In many parts of the world—particularly rural areas or low-income regions—pathology services are scarce. The American Society of Clinical Pathology notes a global shortage of pathologists, with developing nations often having fewer than one per million people. In places like sub-Saharan Africa or rural India, patients might wait months for a cancer diagnosis, if they get one at all, as samples must be shipped to distant labs or cities. For the poor, the cost of such services—travel, testing, and expert fees—can be prohibitive, leaving treatable diseases undetected until it’s too late.
AI could change this equation. Once trained, an AI system’s operational costs plummet compared to human labor. A pathologist’s salary, often exceeding $300,000 annually in the U.S., dwarfs the marginal cost of running an AI algorithm per slide—potentially just a few cents once infrastructure is in place. Digital pathology platforms, where slides are scanned and uploaded to the cloud, allow AI to analyze samples from anywhere with minimal equipment. A small clinic in a remote village could digitize a biopsy and get results from an AI hosted thousands of miles away, bypassing the need for a local specialist.
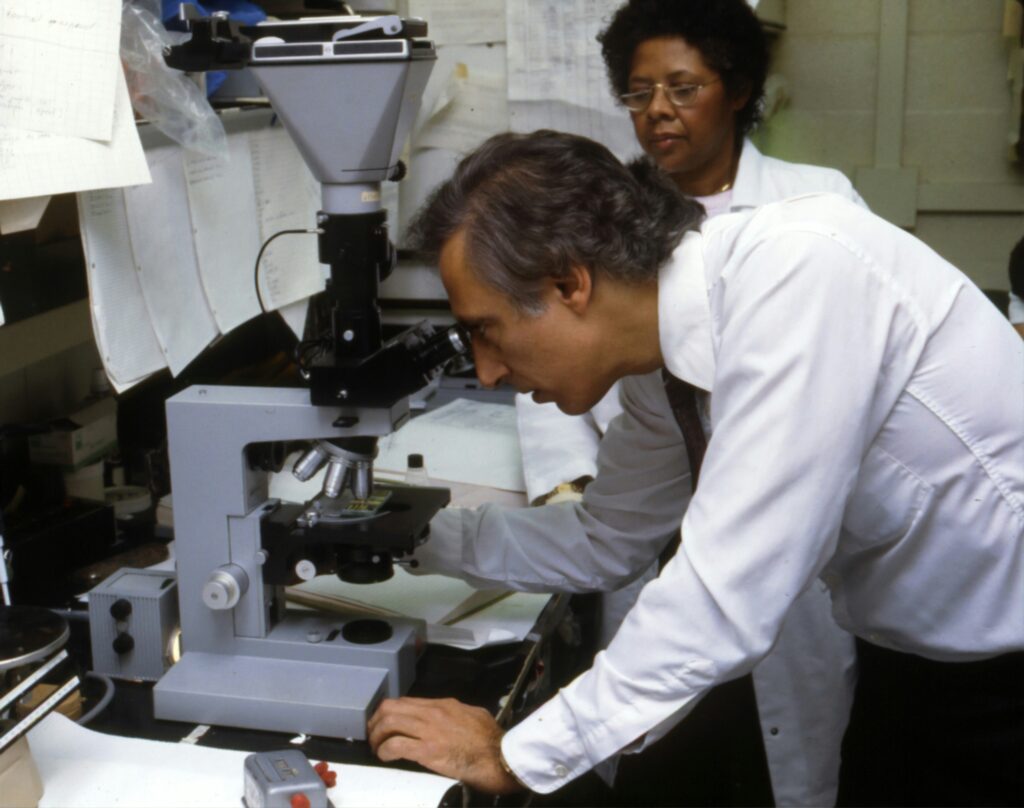
This affordability and reach could bring pathology to the masses. Portable slide scanners, paired with AI, could be deployed in mobile health units or low-budget clinics, serving impoverished urban neighborhoods or isolated rural communities. A farmer in Bangladesh could have a skin lesion biopsied and diagnosed for cancer without leaving his district. A low-income family in Detroit could access a lymphoma screening that was once reserved for those with premium insurance. By slashing costs and geographic barriers, AI in pathology could turn a luxury service into a universal one.
Real-World Wins and Ripple Effects
The impact is already emerging. In 2024, a pilot in rural China used AI to analyze Pap smears for cervical cancer, a disease that disproportionately kills women in poorer regions due to late detection. The AI system processed thousands of samples from underfunded clinics, cutting diagnosis times from weeks to days and reducing costs by 60%. Women who might have faced months of uncertainty—or no screening at all—received timely care, proving AI’s potential to save lives at scale.
In wealthier systems, AI’s efficiency could redirect resources to underserved populations. Public health networks, like the UK’s NHS, could use savings from automated pathology to fund outreach programs, bringing diagnostics to low-income areas. Private labs might lower fees as AI reduces overhead, making tests more affordable for uninsured patients. The ripple effect is a healthcare system where quality pathology isn’t a privilege—it’s a baseline.
Hurdles on the Horizon
AI in pathology isn’t without challenges. The upfront cost of digitizing labs—scanners, software, and reliable internet—could strain poorer regions, requiring global investment to kickstart adoption. Data bias looms large: if AI is trained on samples from one demographic, it might misdiagnose others, potentially harming diverse or minority populations. Regulatory hurdles also slow deployment, as health agencies demand rigorous validation to ensure AI’s reliability. And then there’s the human factor—patients may balk at a machine-only diagnosis, and pathologists face career upheaval, shifting from hands-on analysis to supervisory roles.
But these obstacles have solutions. International aid and tech partnerships could fund infrastructure, while diverse datasets and continuous learning can address bias. Regulatory frameworks are evolving, and hybrid models—where AI flags issues for human confirmation—can ease trust concerns while preserving jobs in a reimagined form. The path forward requires effort, but the destination is worth it.
A Microscope on Equity
AI in pathology is more than a tech upgrade—it’s a catalyst for fairness. By delivering fast, accurate, and affordable diagnostics, it could ensure that a child in a slum, a laborer in a remote town, or a retiree in a struggling city gets the same shot at early detection as anyone else. The efficiency is dazzling, but the access is revolutionary. As AI takes the microscope from human hands, it doesn’t just replace pathologists—it amplifies their reach, turning a specialized field into a global lifeline. For the world’s poorest, that’s a diagnosis of hope.