The field of radiology has long been a cornerstone of modern medicine, providing critical insights into the human body through X-rays, MRIs, CT scans, and more. Traditionally, these images are analyzed by highly trained radiologists—experts who spend years mastering the art of spotting fractures, tumors, or subtle anomalies. But what if artificial intelligence (AI) could step in, not just to assist, but to transform this process entirely? In the realm of radiology and imaging analysis, AI is poised to replace many human tasks, promising unprecedented efficiency and, crucially, broader access to quality healthcare—especially for underserved and economically disadvantaged populations. This is not a distant dream; it’s a shift already underway, with profound implications for patients, doctors, and global health equity.
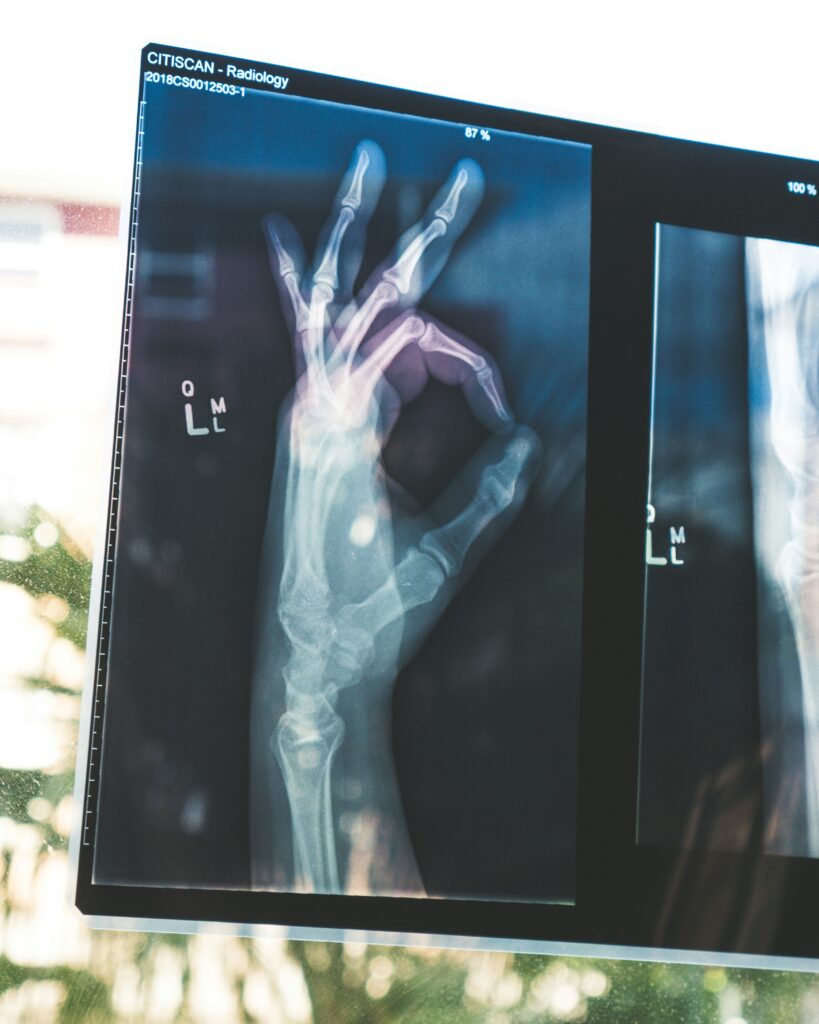
The Efficiency Edge: Speed, Scale, and Precision
One of AI’s most immediate advantages in radiology is its sheer speed. A human radiologist might take anywhere from 10 minutes to an hour to meticulously review a complex scan, depending on the case. AI, by contrast, can process and analyze images in seconds. Advanced algorithms, trained on millions of medical images, can detect patterns—like the telltale signs of pneumonia on a chest X-ray or a brain hemorrhage on a CT scan—faster than the human eye can blink. Companies like Aidoc and Zebra Medical Vision are already deploying AI tools that flag urgent findings in real time, allowing radiologists to prioritize critical cases.
This speed translates to scale. In busy hospitals or clinics, where radiologists are often swamped with hundreds of scans daily, backlogs are common. Patients can wait days or even weeks for results, delaying treatment and increasing anxiety. AI can churn through these queues relentlessly, 24/7, without fatigue or coffee breaks. A study published in The Lancet Digital Health found that AI systems could reduce diagnostic delays by up to 30%, a game-changer for time-sensitive conditions like stroke or cancer.
But it’s not just about speed—AI can also enhance precision. Human radiologists, while skilled, are not infallible. Fatigue, distraction, or the sheer subtlety of some abnormalities can lead to missed diagnoses—studies suggest error rates in radiology hover between 3-5%. AI, when paired with robust training data, can achieve accuracy rates that rival or exceed human performance. For instance, Google’s DeepMind developed an AI that outperformed radiologists in detecting breast cancer from mammograms, reducing false negatives by 9.4%. This precision could mean earlier interventions and better outcomes, particularly for diseases where every day counts.
Bridging the Access Gap: Healthcare for All
While efficiency is impressive, the real revolution lies in how AI could democratize radiology for poorer communities. In many parts of the world—rural areas, developing nations, or underfunded urban clinics—access to skilled radiologists is a luxury. The World Health Organization estimates a global shortage of 4.3 million health workers, with diagnostic specialties like radiology hit especially hard in low-income regions. Patients in these areas might travel hundreds of miles or wait months for a specialist, if they can access one at all. For the economically disadvantaged, the cost of imaging plus expert analysis often puts quality care out of reach.
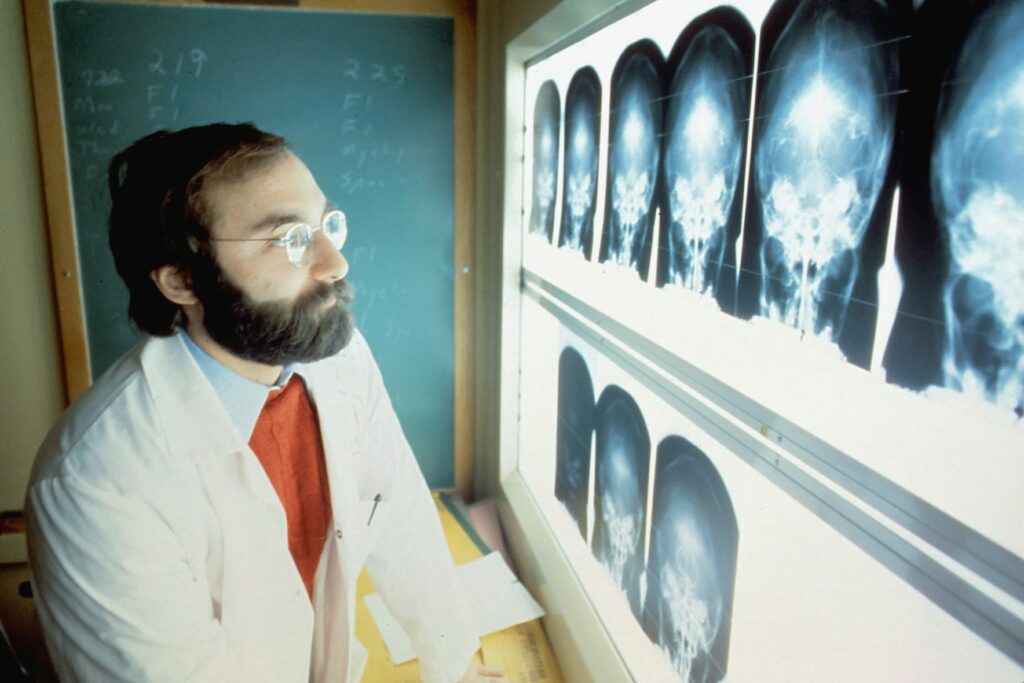
AI promises to flip this script. Once developed and deployed, AI systems can operate at a fraction of the cost of maintaining a full-time radiologist. A single AI platform, hosted in the cloud, could serve multiple hospitals or clinics simultaneously, analyzing scans uploaded from anywhere with an internet connection. Portable imaging devices—like handheld ultrasound machines paired with AI—could bring diagnostics directly to remote villages or impoverished urban centers. Organizations like RadiologyAI are already piloting such solutions in places like sub-Saharan Africa, where AI interprets scans locally, bypassing the need for on-site specialists.
The cost savings are staggering. A radiologist in the U.S. might earn $400,000 annually, while an AI system’s operational costs, once scaled, could drop to pennies per scan. This affordability trickles down to patients, making advanced diagnostics viable for cash-strapped public health systems or uninsured individuals. Imagine a farmer in rural India getting an AI-analyzed X-ray for a broken leg at a local clinic, or a low-income family in Detroit accessing a mammogram screening without a hefty bill. AI doesn’t just streamline radiology—it levels the playing field.
Real-World Impact: Lives Saved, Systems Transformed
The potential for AI to save lives through wider access is already taking shape. In 2023, a pilot program in Rwanda used AI to screen chest X-rays for tuberculosis, a disease that kills over 1.5 million people annually, often in poor communities. The AI system processed scans from mobile clinics, identifying cases in hours rather than weeks, and cut diagnosis costs by 70%. Patients who once might have slipped through the cracks—undiagnosed and untreated—received care sooner, halting the disease’s spread.
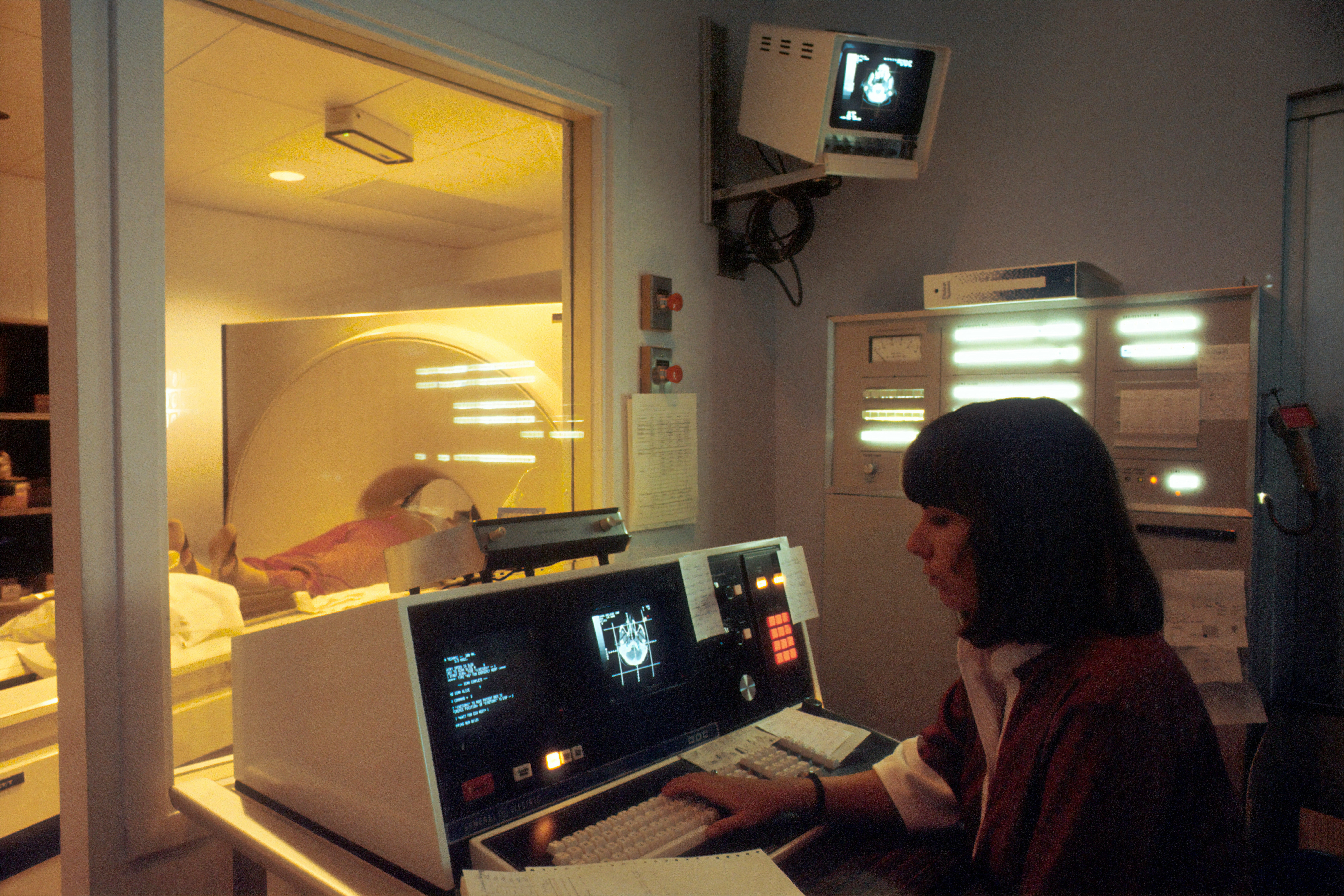
In wealthier nations, AI’s efficiency could free up resources to serve more people. Overburdened healthcare systems, like the U.K.’s NHS, could redirect funds from radiology staffing to other critical areas, such as mental health or preventive care, indirectly benefiting lower-income patients who rely on public services. Even in private systems, reduced costs could lower insurance premiums or out-of-pocket fees, making imaging more accessible across income levels.
Challenges and Caveats
Of course, AI in radiology isn’t a flawless utopia. The tech requires massive upfront investment—building and training these systems isn’t cheap, and poorer regions might struggle to afford the initial infrastructure. Data bias is another hurdle: if AI is trained mostly on images from Western populations, it might falter with diverse patient groups, potentially widening disparities rather than closing them. And then there’s the human element—patients may distrust a machine’s diagnosis without a doctor’s reassuring nod, and radiologists themselves face job displacement, raising ethical questions about livelihoods.
Yet these challenges are not insurmountable. Open-source AI models and international partnerships could lower costs, while diverse datasets and ongoing training can mitigate bias. As for jobs, radiologists might shift from image analysis to oversight and patient care roles, working alongside AI rather than being wholly replaced. The transition won’t be seamless, but the trajectory points toward a net gain for humanity.
A Future Worth Imaging
AI in radiology and imaging analysis is more than a technological leap—it’s a lifeline. By slashing wait times, boosting accuracy, and slashing costs, it could bring world-class diagnostics to the doorsteps of the poor and privileged alike. A single mother in a rural trailer park, a factory worker in Nairobi, or a retiree in a bustling city could all benefit from a system that prioritizes efficiency and equity. While hurdles remain, the promise is clear: AI isn’t just replacing human radiologists—it’s redefining who gets to be seen, diagnosed, and healed. For healthcare’s have-nots, that’s a picture worth more than a thousand words.